Understanding user conversations with one another has moved from the yesteryears’ avenue for merely increasing customer retention or creating segments — to becoming a strategic tool for ‘designing’ community engagements — and thus actively impacting a firm’s growth.
As we evolve in AI — in addition to an evermore data on customer engagements being collected, with a large percentage of this data being customer conversations across a large range of social platforms — reddit, discord, stack, slack, others , the additional opportunity lies in the increasingly nuanced insights that could be drawn and use competitively. Companies which can utilise this data effectively to capture a richer, more nuanced “360 view of Perception” will be setting themselves up for a significant advantage.
However, with the strong momentum towards ‘supervised learning’ within AI, the approach of many AI-enabled systems in extracting such nuances boil down to one of two core ones — A. Labelling conversations with such customer insights, which a modern day LLM can then be fine-tuned to spot, or B. Designing a rules based graph, or pathway of responses such that if the system spots a pre-defined nuance, it would capture that. Notably both approaches are limiting — they not only require large amounts of resources in terms of labelling data and defining structures which both result in a lengthy development time, but additionally — both approaches require explicit definition of what these nuances are and hence the system is not setup to provide any form of new perspectives.
What is Intent?
‘Intent’ is a nuanced insight with a significant value in the customer conversation space. A customer’s intent could be defined as a purpose or goal of a customer when interacting with a peer or an organization. Often, a customer may want a peer or an organization to gain a specific opinion or perform some particular action. For example, a consumer may wish her bank (an organization) to move money from one account to another, or require a new product. which can be then explored with more specific context.
Intents are complex — they may be helpfully explicit — in their most base form consisting of action(s) and an object(s), or quite implicit. An explicit intent would be where the customer directly states what they want, for example, “move money from account X to account Y”. An example of an implicit intent would be “I have lost my debit card” where implicitly the customer wishes to cancel the card and order a new one.
Unsupervised Conversational Insights at Mathlabs
At Mathlabs, our ‘Conversational AI’ methodology helps extract second, third, etc. order customer insights in a wholly un-supervised fashion. The framework is able to ingest conversations on social platforms and extract customer intents without any manual labelling of the data or limiting itself to experts’ list of intents — though results could be filtered further with subject matter experts’ help, post-analysis.
Further, by analysing the contexts in which these nuanced insights appear, enabling early prediction of a nuanced insight could not only reduce the time in directing a customer to the correct service — but actually ‘design’ an optimal customer experience.
A key discovery
Most language AI applications attempt at ‘single-transforms’ i.e. mapping the text space to the target space of nuances (in this case, ‘the space of Intents’) via supervised approaches or prompts to LLMs. At Math Labs, the researchers discovered early that fairly sophisticated insights could be generated — when such an assumption was removed.
We therefore moved to a multi-layer approach, building insights on top of one another — with satisfactory robust results.
Actionability against Customer Discontent
Our approach to extracting Intents today consists of multiple stages, with First the smaller linguistic parts of conversations being transformed into the space of concepts or groups of highly related utterances.
Secondly, we discover component spaces such as Actionability and Discontent — that are key insights in themselves.
Actionability here means, how precisely or tangibly a company is able to respond to a certain customer ask. For example, customers requesting a refund could get more a tangible set of responses compared with customers complaining about being on hold for a long time. Discontent, on the other hand, allows us to judge to what extent the customer is dissatisfied on that ask.
Once the initial concepts are mapped to the space of component insights, we find a range of intents appearing as clusters in this new space.
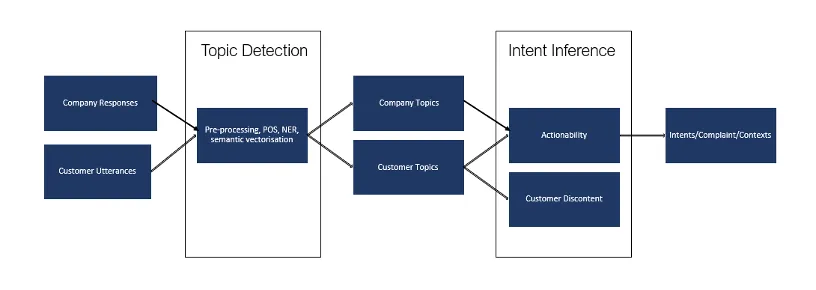
For example — in a relatively trivial case, Actionability and Discontent themselves can be used to discover intent types such as — context, complaints and explicit-intents (or tangible asks) as seen below. Note the top left quadrant of high-actionability low-discontent is left blank because topics rarely fall here (however when they do a possible semantic label could be “Requests”).
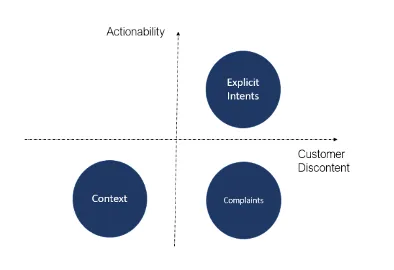
Summary & Utility
The ability to spot new, layered customer nuances such as ‘Intent’ in an unsupervised manner brings many benefits in this new world of socially expressed conversations. Analysis and categorisation of customer conversations with minimal tuning/development time. Extracting insights about customers which are not pre-supposed/ biased by human design. Generating a family of insights — not available earlier — that could be put to use.
Finally, by analysing sequence of the context and complaints which normally precede an explicit intent we can predict a customer’s intent in advance of them stating it, giving us a handle on the elusive implicit intent. By identifying implicit intent, a customer can be served much more effectively with enhanced and enriched experience.